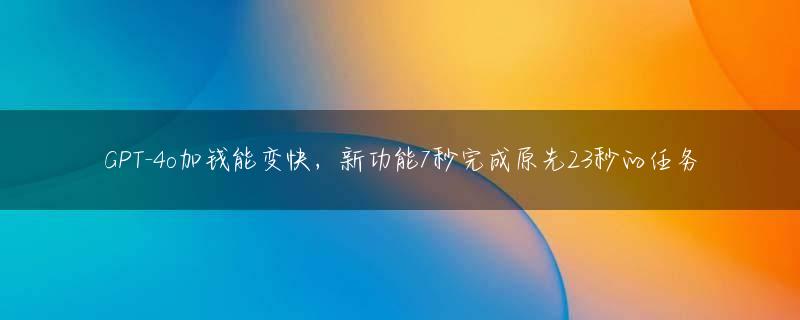
OpenAI出了个新功能,直接让ChatGPT输出的速度原地起飞!
这个功能叫做“预测输出”(Predicted Outputs),在它的加持之下,GPT-4o可以比原先快至多5倍。
以编程为例,来感受一下这个feel:
为啥会这么快?用一句话来总结就是:
跳过已知内容,不用从头开始重新生成。
因此,“预测输出”就特别适合下面这些任务:
在文档中更新博客文章
迭代先前的响应
重写现有文件中的代码
而且与OpenAI合作开发这个功能的FactoryAI,也亮出了他们在编程任务上的数据:
从实验结果来看,“预测输出”加持下的GPT-4o响应时间比之前快了2-4倍,同时保持高精度。
并且官方还表示:
原先需要70秒完成的编程任务,现在只需要20秒。
值得注意的是,目前“预测输出”功能仅支持GPT-4o和GPT-4o mini两个模型,且是以API的形式。
对于开发者而言,这可以说是个利好消息了。
网友们在线实测
消息一出,众多网友也是坐不住了,反手就是实测一波。
例如Firecrawl创始人Eric Ciarla就用“预测输出”体验了一把将博客文章转为SEO(搜索引擎优化)的内容,然后他表示:
速度真的超级快。
它就像在API调用中添加一个预测参数一样简单。
另一位网友则是在已有的代码之上,“喂”了一句Prompt:
change the details to be random pieces of text.将详细信息更改为随机文本片段。
来感受一下这个速度:
也有网友晒出了自己实测的数据:
总而言之,快,是真的快。
怎么做到的?
对于“预测输出”的技术细节,OpenAI在官方文档中也有所介绍。
OpenAI认为,在某些情况下,LLM的大部分输出都是提前知道的。
如果你要求模型仅对某些文本或代码进行细微修改,就可以通过“预测输出”,将现有内容作为预测输入,让延迟明显降低。
例如,假设你想重构一段C#代码,将Username属性更改为Email:
///Represents a user with a first name,last name,and username.
///
publicclassUser
{
///Gets or sets the user's first name.
///
publicstringFirstName{get;set;}
///Gets or sets the user's last name.
///
publicstringLastName{get;set;}
///Gets or sets the user's username.
///
publicstringUsername{get;set;}
}
你可以合理地假设文件的大部分内容将不会被修改(例如类的文档字符串、一些现有的属性等)。
通过将现有的类文件作为预测文本传入,你可以更快地重新生成整个文件。
import OpenAIfrom"openai";
constcode=`
///Represents a user with a first name,last name,and username.
///
publicclassUser
{
///Gets or sets the user's first name.
///
publicstringFirstName{get;set;}
///Gets or sets the user's last name.
///
publicstringLastName{get;set;}
///Gets or sets the user's username.
///
publicstringUsername{get;set;}
}
`;
constopenai=newOpenAI();
constcompletion=awaitopenai.chat.completions.create({
model:"gpt-4o",
messages:[
{
role:"user",
content:"Replace the Username property with an Email property.Respond only with code,and with no markdown formatting."
},
{
role:"user",
content:code
}
],
prediction:{
type:"content",
content:code
}
});
//Inspect returned data
console.log(completion);
使用“预测输出”生成tokens会大大降低这些类型请求的延迟。
不过对于“预测输出”的使用,OpenAI官方也给出了几点注意事项。
首先就是我们刚才提到的仅支持GPT-4o和GPT-4o-mini系列模型。
其次,以下API参数在使用预测输出时是不受支持的:
nvalues greater than 1
logprobs
presence_penaltygreater than 0
frequency_penaltygreater than 0
audiooptions
modalitiesother than text
max_completion_tokens
tools-function calling is not supported
除此之外,在这份文档中,OpenAI还总结了除“预测输出”之外的几个延迟优化的方法。
包括“加速处理token”、“生成更少的token”、“使用更少的输入token”、“减少请求”、“并行化”等等。
文档链接放在文末了,感兴趣的小伙伴可以查阅哦~
One More Thing
虽然输出的速度变快了,但OpenAI还有一个注意事项引发了网友们的讨论:
When providing a prediction,any tokens provided that are not part of the final completion are charged at completion token rates.在提供预测时,所提供的任何非最终完成部分的tokens都按完成tokens费率收费。
有网友也晒出了他的测试结果:
未采用“预测输出”:5.2秒,0.1555美分
采用了“预测输出”:3.3秒,0.2675美分
嗯,快了,也贵了。
OpenAI官方文档:https://platform.openai.com/docs/guides/latency-optimization#use-predicted-outputs
参考链接:[1]https://x.com/OpenAIDevs/status/1853564730872607229[2]https://x.com/romainhuet/status/1853586848641433834[3]https://x.com/GregKamradt/status/1853620167655481411